ABOVE: All-optical deep learning uses 3-D–printed, passive optical components to implement complex functions at the speed of light.
OZCAN LAB @ UCLA
If you want an extremely fast image- or object-recognition system to detect moving items like a missile or cars on the road, a digital camera hooked up to a computer just won’t do, according to electrical engineer Aydogan Ozcan of the University of California, Los Angeles. So, using machine learning, optics tools, and 3-D printing, he and his colleagues have created a system that is more rapid, operates using light and, unlike computers, does not require a power source other than the initial light source and a simple detector. Their results are published today (July 26) in Science.
“This is a very innovative approach to construct a physical artificial neural network made of stacked layers of optical elements,” Demetri Psaltis, a professor of optics and...
What is novel here is not the deep-learning part, but the optical engineering and the ability to “make a cast” of the artificial neural network using 3-D printing, notes Olexa Bilaniuk, a graduate student in Roland Memisevic and Yoshua Bengio’s groups at the University of Montreal who studies machine learning and artificial neural networks. “Previous work to create such an optical network had either been theoretical, or had built much simpler and smaller systems,” he adds.
Ozcan would like to use the system to mimic various animal eyes, which process light and images differently than the human eye. The invention could also be used for microscopy applications and medical imaging, he says, if implemented at the shorter wavelengths used in optical microscopy.
To build their recognition system, Ozcan and his colleagues first used an approach known as deep learning, in which a computer is given audio or visual data and trained to recognize certain patterns. The algorithm then creates rules about the given data and applies them to describe new inputs.
In this case, the team trained its network model to recognize different data types, including images of hand-written digits from 0 to 9 and various clothing items. In each case, the computer created a model, which consisted of multiple layers of pixels. Each pixel can transmit light, and represents an artificial neuron connected to other neurons in the same or attached layers.
For each data type, the researchers then made a physical rendition of the model using five layers of a 3-D–printed plastic that could then process each type of image using a laser—a monochromatic light at 0.4 terahertz—rather than visible light.
The printed polymer neural network takes in the light bouncing off an object and processes it at the speed of light based on the physical structure of the neural network and rapidly sorts the object or its image into the correct category by focusing light onto the correct detector at the exit of the network.
The printed product is like a “physical brain with neural connections, except here, light is connecting the neurons such that the information can flow from one layer of the neurons to the next,” explains Ozcan.
“This is an extremely efficient neural network implementation, because once the passive diffraction surfaces are 3D-printed, they use no electricity whatsoever but process their input ‘at the speed of light’, with no delay,” writes Bilaniuk in an email to The Scientist.
The researchers are now working to improve the performance of the computer-training model. In the case of the digits training set, their artificial network had about a 91.75 percent accuracy in recognizing new handwritten digits. They would also like to scale their printed network to larger dimensions than the printed 8 cm by 8 cm layers reported in the paper. “With more layers, we can potentially implement more-complex tasks with improved accuracy,” Ozcan tells The Scientist.
For Psaltis, the new work poses more questions than it answers. “Can this system be made more robust, can we increase the speed, what is the cost of the modeling and the 3-D printing, and how could we potentially integrate this tool with existing digital systems?” he writes.
According to Bilaniuk, if the system could be adapted for regular light and miniaturized, the potential applications could be face detection in cell phone cameras and automatic focus with no drain on the battery as is necessary for digital devices.
X. Lin et al., “All-optical machine learning using diffractive deep neural networks,” Science, doi:10.1126/science.aat8084, 2018.
Interested in reading more?
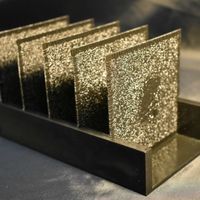